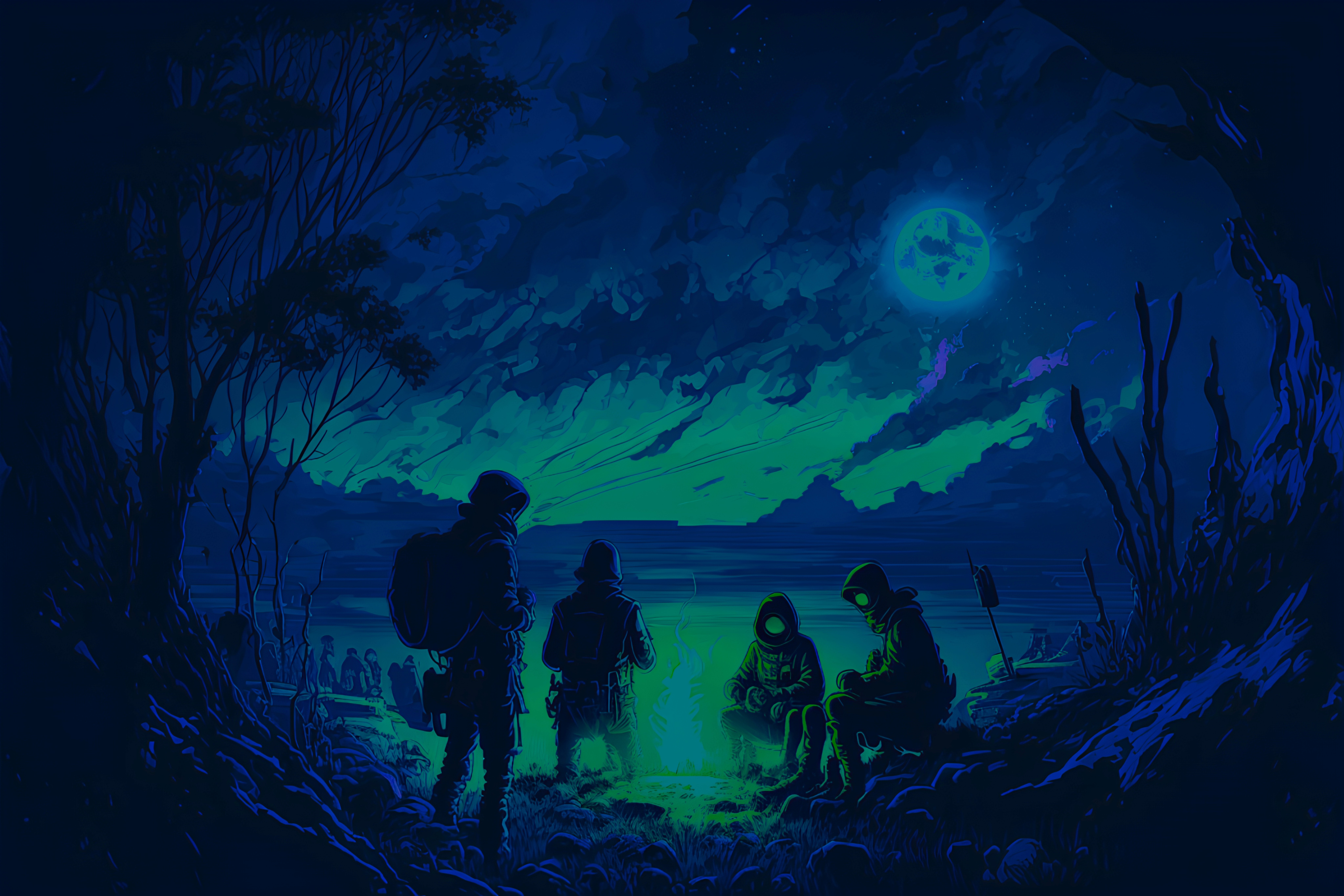
Business
ML/AI
How to do customer research with chatGPT

Shavin
•
Jan 4, 2023
Doing customer research for your idea or startup just became a whole lot easier. Introducing a simple chatGPT workflow that can 2x your output.
I recently figured out how to use chatGPT (an experimental beta of a large language model or LLM created by OpenAI) to make my customer research workflow twice as fast.
Like most product designers, I spend a lot of time talking to customers to identify and understand their needs.
My latest project was with a home construction company that wanted to position itself better in the market. These kinds of projects tend to start off slow because of the time it takes to conduct surveys and interviews, then analyze and present the results.
In the next 10 min, I’ll walk you through the exact step-by-step process I used to create a workflow with chatGPT that enabled me to work twice as fast.
If you haven't played around with chatGPT before, I highly recommend going over the below for some tl;dr:
Alright, here's quick breakdown of what we'll cover:
Current research methodology
Organizing quotes into patterns
Synthesizing information
Taking it further
Buckle in!
The process I have used for years is pretty straight forward. For example, a recent project I worked on was in the construction domain. This is what I did:
I interviewed 9 people who'd recently made the journey to build a house.
I transcribed those conversations by capturing key quotes with timestamps.
Then I organized the individual conversations from the interviews into specific themes or categories, and then counted the number of times a quote was mentioned in each theme.
Finally, I synthesized the findings for presentation.
Usually, the most "automated" part of this process was the use of otter.ai to transcribe the conversations.
The rest is usually spent in Apple Notes tediously organizing quotes and synthesizing them.
This can take anywhere between 2-3 days to get to actionable learnings without missing out on anything. As much as I love talking to customers, this was a painful but important part of the process. Well… until chatGPT.
So let's find out what we can achieve with chatGPT.
Organizing quotes into patterns
I usually conduct interviews with JTBD (Jobs to be done). You can read more about it here if you want to dive deeper. These interviews are never linear, the conversations tend to jump back and forth in the buyers timeline so I have to take a bottom-up approach.
INPUT PROMPT: Group these customer quotes into crisp, actionable themes. Include the number of times a quote appears for each theme, who said the quote, and a snippet of the quote relating to the theme. Do it in the table format.
Tasking it to theme the customer quotes that got people started on the journey to build a house. Here's what it gave me back:
Customer quotes organised by theme. 🤯
I experimented with it a lot and found through trial and error that if your input prompt is too broad, the output often suffers drastically. ChatGPT can't decide which level of abstraction to theme it. So you have to be very specific.
One benefit of using Chat GPT for organizing themes is that you can ask it to refine its output by providing additional input.
It's like having a helpful coworker who can iterate on your feedback almost instantly. In my example, I had to ask it for "crisp and actionable" themes, and it was able to generate the result you see above.
One potential downside of using Chat GPT like this is that if you input the same prompt and quotes multiple times, it may categorize them differently each time.
For example, a quote filed under "personal satisfaction" might be grouped under "desire for ownership" on a different, subsequent run. This lack of consistency can make it difficult to use effectively in a team setting.
⚠️ DATA LIMITS
There is a load limit in the amount of data you can feed chatGPT. For example, if you have a CSV with 10,000 quotes, chatGPT will give you an error.
To combat this, just reduce your rows to 40-50.
Synthesizing information
Synthesizing information is both an art and a science. Quotes from an interview don't include the context or the underlying emotions of what was said, which can lead to different researchers interpreting the same quote in different ways.
So, how does Chat GPT handle this?
Synthesizing job stories
Before chatGPT, I used to synthesize the quotes into job stories and then write a summary presenting all the findings.
I personally struggled a lot with this step as it requires careful thought and phrasing in order to communicate the situation and progress customers are looking for.
Now with chatGPT, I am able to get desirable outputs with prompt chaining.
I wanted to generate stories for people who have built a house. But, there was a small issue. When you ask it to generate the job stories using individual quotes, it tries to do it for each user rather than creating stories across the group. So, instead, you have to ask it to create the high level themes first.
This is where prompt chaining comes into play.
Let's walk through the example:
INPUT PROMPT 1: Group these customer quotes into themes for wanting to build a house.[Paste the quotes]And after the results are shown...
INPUT PROMPT 2: Now could you write them in the job stories format starting with When I...?
This output was fairly decent. It was even able to produce the second job story which was a key focus in the research that I had conducted. That's a win!
However, it does make some assumptions which can threaten the integrity of the research. In point 2, it writes about not being constrained by the landlord but there was no mention of it in the quotes or interviews as an outcome that they wanted to achieve. It's a plausible outcome in real life but it didn't show up in the research data.
Furthermore, some of the stories don't frame the situation customers are in properly.
For example, "When I have savings that I want to invest in a way that will appreciate in value" sounds more like a motivation than a situation. In my research, I framed this as "When my earnings is depreciating in a savings/fixed deposit account" which is a situation that you can design for.
🌋
Share your journey so far
Further synthesis of marketing & service-related challenges
The final step in my research process involves analyzing all the data and identifying challenges that my clients would have to address at the top and middle of the customer journey.
This is the point where customers are discovering the business and deciding whether or not to engage with it.
ChatGPT is useful here too. I asked it to take the customer quotes and create some problem statements in the HMW (how might we) format. HMW's are a design thinking tool to reframe problems as opportunities.The best use of this is right before the brainstorming stage.
INPUT PROMPT: Generate 5 problem statements in the HMW format so that we can be discovered by these types of customers
Not bad! Each of those challenges broadly targets different customer motivations with some healthy overlap.
My clients hired me to identify these challenges. I got them in a matter of seconds as opposed to multiple hours worth of work. So now we can go from interviews to insights very quickly.
Let's say we solved some of those challenges and attracted some leads. What sort of challenges would we have to address to convert them into customers?
INPUT PROMPT: Generate 5 problem statements in the HMW format so that we can convert these prospects into customers
Alright, that's pretty ridiculous. When I tried this for the first time, I was actually shaken by the output.
Mainly because I didn't feed it any quotes around what service attributes customers liked about the company that they choose to go with. It's generating these based only on the data it has related to customers struggling moments.
Additionally, the problem statements are neither too narrow or broad. They're at just the right level to get ideas flowing.
Despite some limitations in its ability to organize data properly, it was able to generate relevant challenges for prioritization and ideation. Hallelujah!
Finally, I asked it to develop some service related challenges that we'd have to solve for, in order to provide a great customer experience.
INPUT PROMPT: Generate 5 problem statements in the HMW format that we need to execute so that we can provide a great customer experience
Taking it even further
Alright, I know the note is a bit long and you just wanna go try this out. But bear with me for a couple more minutes.
From conducting the research interviews, it was clear to me that the segment of customers who had the job of wanting "a space of their own to live with their partner and avoid conflicts with parents" was a viable target segment to go after first.
In Sri Lanka, it's normal for couples to move in with parents after marriage. But as couples mature through time, the need for having their own space, independence and freedom to do things their own way increases. This causes them to switch to a new way of making progress. In our case, this meant building a house.
I never got to this stage with my clients but it made me wonder about what sort of low cost marketing strategy could it suggest that's specific to this segment of customers.
Can chatGPT help me with create a marketing strategy to target these folks? Let's find out.
INPUT PROMPT: Generate a low cost marketing strategy to target couples who are looking to gain more independence from parents
These are great! I could go even deeper by asking it questions around each suggestion. But this just shows you the potential of having a tool like this in your backpocket!
Final thoughts
When I started exploring Chat GPT's potential application for streamlining research, I didn't expect this rollercoaster of emotions. It's clear that it's a powerful tool for synthesizing challenges and ideas when it's grounded by customer quotes. It's not perfect as it can definitely improve over time the ability to organize and synthesize data. What's clear right now is how much time it can potentially save me.
In the future, I can definitely imagine the ideal "AI driven" research tool being somewhat like an oracle. You give it raw customer data (quantitative quotes and qualitative metrics), and anyone in your team can query it on what their customers struggle with and gain answers on how to alleviate those frustrations. There won't be a need to code findings since the AI can theme it for you.
Finally, be aware that since it's still in the research preview stage, it isn't as reliable for heavy use. I encourage that you use it as a sounding board for discussing and ideating your research at the moment. Eventually, they'll make it better for all of us to use.
Good luck and have fun!
Shavin